Informatica, IBM Datastage, SAP, Oracle & SAS-Dataflux are in the Leader's quadrant. Microsoft in the challenger quadrant followed by Syncsort, Talend, Pervasive Software and Information builder.
Sunday, December 9, 2012
Wednesday, November 28, 2012
Gartner: Top 10 Strategic Technology Trends For 2013
ü Mobile Devices
ü Mobile Application
ü Personal Cloud
ü internet and things
ü hybrid IT & Cloud Computing
ü Strategic Big data
ü Actionable analytics
ü mainstream In-Memory Computing
ü integrated Ecosystems
ü Enterprise app Stores
The Forrester Wave™: Enterprise Cloud Databases, Q4 2012
Forrester conducted product evaluations and interviewed six vendor companies: Amazon Web Services (AWS), Caspio, EnterpriseDB, Microsoft, salesforce. com, and Xeround.
Microsoft, two products from Amazon Web Services (AWS) — Amazon Relational Database Service (RDS) and Amazon DynamoDB — and salesforce.com’s Database.com as leading the pack due to their breadth of cloud database capabilities and strong product strategy and vision. EnterpriseDB, salesforce.com’s Heroku Postgres, Caspio, and Xeround are close on the heels of the Leaders, offering viable solutions to support most current cloud database requirements.
Wednesday, August 8, 2012
The Hype Cycle for Cloud Computing, 2012 by Gartner
Here are few of the highlights “The Hype Cycle for Cloud Computing, 2012 by Gartner” published in Forbes.
o The Cloud BPM (bpmPaaS) market is slated to grow 25% year over year, and 40% of companies doing BPM are already using BPM in the cloud.
o Cloud Email is expected to have a 10% adoption rate in enterprises by 2014, down from the 20% Gartner had forecasted in previous Hype Cycles.
o Big Data will deliver transformational benefits to enterprises within 2 to 5 years, and by 2015 will enable enterprises adopting this technology to outperform competitors by 20% in every available financial metric.
o Master Data Management (MDM) Solutions in the Cloud and Hybrid IT are included in this hype cycle for the first time in 2012.
o PaaS continues to be one of the most misunderstood aspects of cloud platforms.
o By 2014 the Personal Cloud will have replaced the personal computer as the center of user’s digital lives.
o Private Cloud Computing is among the highest interest areas across all cloud computing according to Gartner, with 75% of respondents in Gartner polls saying they plan to pursue a strategy in this area by 2014.
o SaaS is rapidly gaining adoption in enterprises, leading Gartner to forecast more than 50% of enterprises will have some form of SaaS-based application strategy by 2015.
o More than 50% of all virtualization workloads are based on the x86 architecture.
For More Refer: http://www.forbes.com/sites/louiscolumbus/2012/08/04/hype-cycle-for-cloud-computing-shows-enterprises-finding-value-in-big-data-virtualization/
Monday, July 23, 2012
The Forrester Wave™: Advanced Data Visualization (ADV) Platforms, Q3 2012
Summary: Enterprises find advanced data visualization (ADV) platforms to be essential tools that enable them to monitor business, find patterns, and take action to avoid threats and snatch opportunities. In Forrester’s 29-criteria evaluation of ADV vendors, we found that Tableau Software, IBM, Information Builders, SAS, SAP, Tibco Software, and Oracle led the pack due to the breadth of their ADV business intelligence (BI) functionality offerings. Microsoft, MicroStrategy, Actuate, QlikTech, Panorama Software, SpagoBI, Jaspersoft, and Pentaho were close on the heels of the Leaders, also offering solid functionality to enable business users to effectively visualize and analyze their enterprise data.
Tuesday, July 17, 2012
The Forrester Wave™: Self-Service Business Intelligence Platforms, Q2 2012
Summary: In Forrester’s 31-criteria evaluation of self-service business intelligence (BI) vendors, we found that IBM, Microsoft, SAP, SAS, Tibco Software, and MicroStrategy led the pack due to the breadth of their selfservice BI functionality offerings. Information Builders, Tableau Software, Actuate, Oracle, QlikTech, and Panorama Software were close on the heels of the Leaders, also offering solid functionality to enable business users to self-serve most of their BI requirements.
Thursday, July 5, 2012
ETL Tool Evaluation Criteria
There are various ETL tools in the market such as Informatica, IBM DataStage, AbInitio, SAP BODI, Pentaho Kettel, Microsoft SSIS, Oracle ODI.. etc. Finalizing the righ data integration tool is critical success factor for any Organization.
ETL Tool Evaluation is based on following Parameters
• Architecture
• Metadata Support
• Ease of Support
• Transformations
• Performance /Management
• Data Quality & MDM
• Support for Growth
• Advance Data Transformation
• 3rd Party Compatibility
• License and Pricing
• Vendor Information
ETL Tool Evaluation is based on following Parameters
• Architecture
• Metadata Support
• Ease of Support
• Transformations
• Performance /Management
• Data Quality & MDM
• Support for Growth
• Advance Data Transformation
• 3rd Party Compatibility
• License and Pricing
• Vendor Information
For more detail Please refer mentioned below link:
Thursday, June 28, 2012
Hadoop Implementation for Big Data
Myth #1: Big Data is Only About Massive Volume.
Myth #2: Big Data Means Hadoop.
Myth #3: Big Data Means Unstructured Data.
Myth #4: Big Data is for Social Media Feeds and Sentiment Analysis.
Myth #5: NoSQL means No SQL.
Here is one holistic view of Big Data Implementation.
Wednesday, June 6, 2012
Thursday, May 24, 2012
Current trends affecting predictive analytic
I was going through one article by Johan Blomme on predictive analytic and found really interesting. Here is a lil summary:
Traditionally, BI systems provided a retrospective view of the business by querying data warehouses containing historical data. Contrary to this, contemporary BI-systems analyze real-time event streams in memory. In today’s rapidly changing business environment, organizational agility not only depends on operational monitoring of how the business is performing but also on the prediction of future outcomes which is critical for a sustainable competitive position.
Predictive analytics leverages actionable intelligence that can be integrated in operational processes.
Current trends affecting predictive analytic:
· Standards for Data mining and Model Deployment
· Predictive Analytics in the Cloud
· Structured and Un Structured Data types
· Advance Database Technology (MPP, Column Based, In Memory..etc)
Standards for data mining and model deployment : CRISP-DM
o A systematic approach to guide the data mining process has been developed by a consortium of vendor and users of data mining, known as Cross Industry Standard for Data Mining (CRISP-DM).
o In the CRISP-DM model, data mining is described as an interactive process that is depicted in several phases (business and data understanding, data preparation, modeling, evaluation and deployment) and their respective tasks. Leading vendors of analytical software offer workbenches that make the CRISP-DM process explicit.
Standards for data mining and model deployment : PMML
o To deliver a measurable ROI, predictive analytics requires a focus on decision optimization to achieve business objectives. A key element to make predictive analytics pervasive is the integration with commercial lines operations. Without disrupting these operations, business users should be able to take advantage of the guidance of predictive models.
o For example, in operational environments with frequent customer interactions, high-speed scoring of real-time data is needed to refine recommendations in agent-customer interactions that address specific goals, e.g. improve retention offers. A model deployed for these goals acts as a decision engine by routing the results of predictive analytics to users in the form of recommendations or action messages.
o A major development for the integration of predictive models in business applications is the PMML-standard (Predictive Model Markup Language) that separates the results of data mining from the tools that are used for knowledge discovery.
Structured and unstructured data types:
o The field of advanced analytics is moving towards providing a number of solutions for the handling of big data. Characteristic for the new marketing data is its text-formatted content in unstructured data sources which covers « the consumer’s sphere of influence » : analytics must be able to capture and analyze consumer-initiated communication.
o By analyzing growing streams of social media content and sifting through sentiment and behavioral data that emanates from online communities, it is possible to acquire powerful insights into consumer attitudes and behavior. Social media content gives an instant view of what is taking place in the ecosystem of the organization. Enterprises can leverage insights from social media content to adapt marketing, sales and product strategies in an agile way.
o The convergence between social media feeds and analytics also goes beyond the aggregate level. Social network analytics enhance the value of predictive modeling tools and business processes will benefit from new inputs that are deployed. For example, the accuracy and effectiveness of predictive churn analytics can be increased by adding social network information that identifies influential users and the effects of their actions on other group members.
Advances in database technology : big data and predictive analytics
o As companies gather larger volumes of data, the need for the execution of predictive models becomes more prevalent.
o A known practice is to build and test predictive models in a development environment that consists of operational data and warehousing data. In many cases analysts work with a subset of data through sampling. Once developed, a model is copied to a runtime environment where it can be deployed with PMML. A user of an operational application can invoke a stored predictive model by including user defined functions in SQL-statements. This causes the RDBMS to mine the data iself without transferring the data into a separate file. The criteria expressed in a predictive model can be used to score, segment, rank or classify records.
o An emerging practice to work with all data and directly deploy predictive models is in-database analytics. For example, Zementis (www.zementis.com) and Greenplum (www.greenplum.com) have joined forces to score huge amounts of data in-parallel. The Universal PMLL Plug-in developed by Zementis is an in-database scoring engine that fully supports the PMML-standard to execute predictive models from commerial and open source data mining tools within the database.
Predictive analytics in the cloud
o While vendors implement predictive analytics capabilities into their databases, a similar development is taking place in the cloud. This has an impact on how the cloud can assist businesses to manage business processes more efficiently and effectively. Of particular importance is how cloud computing and SaaS provide an infrastructure for the rapid development of predictive models in combination with open standards. The PMML standard has yet received considerable adoption and combined with a service-oriented architecture for the design of loosely coupled systems, the cloud computing/SaaS model offers a cost-effective way to implement predictive models.
o As an illustration of how predictive models can be hosted in the cloud, we refer to the ADAPA scoring engine (Adaptive Decision and Predictive Analytics, www.zementis.com). ADAPA is an on demand predictive analytics solution that combines open standards and deployment capabilities. The data infrastructure to launch ADAPA in the cloud is provided by Amazon Web Services (www.amazonwebservices.com). Models developed with PMML-compliant software tools (e.g. SAS, Knime, R, ..) can be easily uploaded in the ADAPA environment.
o The on-demand paradigm allows businesses to use sophisticated software applications over the Internet, resulting in a faster time to production with a reduction of total cost of ownership.
o Moving predictive analytics into the cloud also accelerates the trend towards self-service BI. The so-called democratization of data implies that data access and analytics should be available across the enterprise. The fact that data volumes are increasing as well as the need for insights from data, reinforce the trend for self-guided analysis. The focus on the latter also stems from the often long development backlogs that users experience in the enterprise context. Contrary to this, cloud computing and Saas enable organizations to make use of solutions that are tailored to specific business problems and complement existing systems.
Tuesday, May 22, 2012
Next Generation MDM (From TDWI)
What is MDM (Master Data Management)?
Master data management (MDM) is the practice of defining and maintaining consistent definitions of business entities (e.g., customer or product) and data about them across multiple IT systems and possibly beyond the enterprise to partnering businesses. MDM gets its name from the master and/or reference data through which consensus-driven entity definitions are usually expressed. An MDM solution provides shared and governed access to the uniquely identified entities of master data assets, so those enterprise assets can be applied broadly and consistently across an organization.
Top 10 Priorities for Next Generation MDM
1. Multi-data-domain MDM Many organizations apply MDM to the customer data domain alone,and they need to move on to other domains, such as products, financials, and locations. Singledata-domain MDM is a barrier to correlating information across multiple domains.
2. Multi-department, multi-application MDM MDM for a single application (such as ERP, CRM,or BI) is a safe and effective start. But the point of MDM is to share data across multiple,diverse applications and the departments that depend on them. It’s important to overcomeorganizational boundaries if MDM is to move from being a local fix to being an infrastructurefor sharing data as an enterprise asset.
3. Bidirectional MDM “Roach motel” MDM is when you extract reference data and aggregate it in a master database from which it never emerges (as with many BI and CRM systems). Unidirectional MDM is fine for profiling reference data, but bidirectional MDM is required to improve or author reference data in a central place and then publish it out to various applications.
4. Real-time MDM The strongest trend in data management today (and BI/DW, too) is toward realtime operation as a complement to batch. Real time is critical to verification, identity resolution, and the immediate distribution of new or updated reference data.
5. Consolidating multiple MDM solutions How can you create a single view of the customer when you have multiple customer-domain MDM solutions? How can you correlate reference data across domains when the domains are treated in separate MDM solutions? For many organizations, next generation MDM begins with a consolidation of multiple, siloed MDM solutions.
6. Coordination with other disciplines To achieve next generation goals, many organizations need to stop practicing MDM in a vacuum. Instead of MDM as merely a technical fix, it should also align with business goals for data. MDM should also be coordinated with related data management disciplines, especially DI and DQ. A program for data governance or stewardship can provide an effective collaborative process for such coordination.
7. Richer modeling Reference data in the customer domain works fine with flat modeling, involving a simple (but very wide) record. However, other domains make little sense without a richer, hierarchical model, as with a chart of accounts in finance or a bill of materials in manufacturing. Metrics and key performance indicators—so common in BI, today—rarely have proper master data in multidimensional models.
8. Beyond enterprise data Despite the obsession with customer data that most MDM solutions suffer, almost none of them today incorporate data about customers from Web sites or social media. If you’re truly serious about MDM as an enabler for CRM, next generation MDM (and CRM, too) must reach into every customer channel. In a related area, users need to start planning their strategy for MDM with big data and advanced analytics.
9. workflow and process management Too often, development and collaborative efforts in MDM are mostly ad hoc actions with little or no process. For an MDM program to scale and grow, it needs workflow functionality that automates the proposal, review, and approval process for newly created or improved reference data. Vendor tools and dedicated applications for MDM now support workflows within the scope of their tools. For a broader scope, some users integrate MDM with BPM tools.
10. MDM solutions built atop vendor tools and platforms Admittedly, many user organizations find that homegrown and hand-coded MDM solutions provide adequate business value and technical robustness. However, these solutions are usually in simple departmental silos. User organizations should look into vendor tools and platforms for MDM and other data management disciplines when they need broader data sharing and more advanced functionality, such as real-time operation, two-way synchronization, identity resolution, event processing, service orientation, and process workflows or other collaborative functions.
Wednesday, March 21, 2012
Busting 10 Myths about Hadoop By Philip Russom (TDWI)
Fact #1. Hadoop consists of multiple products.
We talk about Hadoop as if it’s one monolithic thing, whereas it’s actually a family of open-source products and technologies overseen by the Apache Software Foundation (ASF). (Some Hadoop products are also available via vendor distributions; more on that later.)
The Apache Hadoop library includes (in BI priority order): the Hadoop Distributed File System (HDFS), MapReduce, Hive, Hbase, Pig, Zookeeper, Flume, Sqoop, Oozie, Hue, and so on. You can combine these in various ways, but HDFS and MapReduce (perhaps with Hbase and Hive) constitute a useful technology stack for applications in BI, DW, and analytics.
Fact #2. Hadoop is open source but available from vendors, too.
Apache Hadoop’s open-source software library is available from ASF at http://www.apache.org. For users desiring a more enterprise-ready package, a few vendors now offer Hadoop distributions that include additional administrative tools and technical support.
Fact #3. Hadoop is an ecosystem, not a single product.
In addition to products from Apache, the extended Hadoop ecosystem includes a growing list of vendor products that integrate with or expand Hadoop technologies. One minute on your favorite search engine will reveal these.
Fact #4. HDFS is a file system, not a database management system (DBMS).
Hadoop is primarily a distributed file system and lacks capabilities we’d associate with a DBMS, such as indexing, random access to data, and support for SQL. That’s okay, because HDFS does things DBMSs cannot do.
Fact #5. Hive resembles SQL but is not standard SQL.
Many of us are handcuffed to SQL because we know it well and our tools demand it. People who know SQL can quickly learn to hand-code Hive, but that doesn’t solve compatibility issues with SQL-based tools. TDWI feels that over time, Hadoop products will support standard SQL, so this issue will soon be moot.
Fact #6. Hadoop and MapReduce are related but don’t require each other.
Developers at Google developed MapReduce before HDFS existed, and some variations of MapReduce work with a variety of storage technologies, including HDFS, other file systems, and some DBMSs.
Fact #7. MapReduce provides control for analytics, not analytics per se.
MapReduce is a general-purpose execution engine that handles the complexities of network communication, parallel programming, and fault-tolerance for any kind of application that you can hand-code – not just analytics.
Fact #8. Hadoop is about data diversity, not just data volume.
Theoretically, HDFS can manage the storage and access of any data type as long as you can put the data in a file and copy that file into HDFS. As outrageously simplistic as that sounds, it’s largely true, and it’s exactly what brings many users to Apache HDFS.
Fact #9. Hadoop complements a DW; it’s rarely a replacement.
Most organizations have designed their DW for structured, relational data, which makes it difficult to wring BI value from unstructured and semistructured data. Hadoop promises to complement DWs by handling the multi-structured data types most DWs can’t.
Fact #10. Hadoop enables many types of analytics, not just Web analytics.
Hadoop gets a lot of press about how Internet companies use it for analyzing Web logs and other Web data. But other use cases exist. For example, consider the big data coming from sensory devices, such as robotics in manufacturing, RFID in retail, or grid monitoring in utilities. Older analytic applications that need large data samples -- such as customer base segmentation, fraud detection, and risk analysis -- can benefit from the additional big data managed by Hadoop. Likewise, Hadoop’s additional data can expand 360-degree views to create a more complete and granular view.
We talk about Hadoop as if it’s one monolithic thing, whereas it’s actually a family of open-source products and technologies overseen by the Apache Software Foundation (ASF). (Some Hadoop products are also available via vendor distributions; more on that later.)
The Apache Hadoop library includes (in BI priority order): the Hadoop Distributed File System (HDFS), MapReduce, Hive, Hbase, Pig, Zookeeper, Flume, Sqoop, Oozie, Hue, and so on. You can combine these in various ways, but HDFS and MapReduce (perhaps with Hbase and Hive) constitute a useful technology stack for applications in BI, DW, and analytics.
Fact #2. Hadoop is open source but available from vendors, too.
Apache Hadoop’s open-source software library is available from ASF at http://www.apache.org. For users desiring a more enterprise-ready package, a few vendors now offer Hadoop distributions that include additional administrative tools and technical support.
Fact #3. Hadoop is an ecosystem, not a single product.
In addition to products from Apache, the extended Hadoop ecosystem includes a growing list of vendor products that integrate with or expand Hadoop technologies. One minute on your favorite search engine will reveal these.
Fact #4. HDFS is a file system, not a database management system (DBMS).
Hadoop is primarily a distributed file system and lacks capabilities we’d associate with a DBMS, such as indexing, random access to data, and support for SQL. That’s okay, because HDFS does things DBMSs cannot do.
Fact #5. Hive resembles SQL but is not standard SQL.
Many of us are handcuffed to SQL because we know it well and our tools demand it. People who know SQL can quickly learn to hand-code Hive, but that doesn’t solve compatibility issues with SQL-based tools. TDWI feels that over time, Hadoop products will support standard SQL, so this issue will soon be moot.
Fact #6. Hadoop and MapReduce are related but don’t require each other.
Developers at Google developed MapReduce before HDFS existed, and some variations of MapReduce work with a variety of storage technologies, including HDFS, other file systems, and some DBMSs.
Fact #7. MapReduce provides control for analytics, not analytics per se.
MapReduce is a general-purpose execution engine that handles the complexities of network communication, parallel programming, and fault-tolerance for any kind of application that you can hand-code – not just analytics.
Fact #8. Hadoop is about data diversity, not just data volume.
Theoretically, HDFS can manage the storage and access of any data type as long as you can put the data in a file and copy that file into HDFS. As outrageously simplistic as that sounds, it’s largely true, and it’s exactly what brings many users to Apache HDFS.
Fact #9. Hadoop complements a DW; it’s rarely a replacement.
Most organizations have designed their DW for structured, relational data, which makes it difficult to wring BI value from unstructured and semistructured data. Hadoop promises to complement DWs by handling the multi-structured data types most DWs can’t.
Fact #10. Hadoop enables many types of analytics, not just Web analytics.
Hadoop gets a lot of press about how Internet companies use it for analyzing Web logs and other Web data. But other use cases exist. For example, consider the big data coming from sensory devices, such as robotics in manufacturing, RFID in retail, or grid monitoring in utilities. Older analytic applications that need large data samples -- such as customer base segmentation, fraud detection, and risk analysis -- can benefit from the additional big data managed by Hadoop. Likewise, Hadoop’s additional data can expand 360-degree views to create a more complete and granular view.
Tuesday, March 6, 2012
The Forrester Wave™ Enterprise ETL, Q1 2012

Data movement is critical in any organization to support data management initiatives, such as data warehousing (DW), business intelligence (BI), application migrations and upgrades, master data management (MDM), and other initiatives that focus on data integration. Besides moving data, ETL supports complex transformations like cleansing, reformatting, aggregating, and converting very large volumes of data from many sources. In a mature integration architecture, ETL complements change data capture (CDC) and data replication technologies to support real-time data requirements and combines with application integration tools to support messaging and transactional integration. Although ETL is still used extensively to support traditional scheduled batch data feeds into DW and BI environments, the scope of ETL has evolved over the past five years to support new and emerging data management initiatives, including:
- Data virtualization
- Cloud integration
- Big Data
- Real-time data warehousing
- Data migration and application retirement
- Master data management
IBM Datastage, Informatica, Oracle (Oracle Data Integration - ODI & Oracle Warehouse Builder - OWB), SAP (BusinessObjects’ Data Integrator - BODI), SAS, Ab Initio, And Talend Lead, With Pervasive, Microsoft (SSIS), And iWay Close Behind in The Forrester Wave™ Enterprise ETL, Q1 2012.
IBM Datastage, Informatica, Oracle (Oracle Data Integration - ODI & Oracle Warehouse Builder - OWB), SAP (BusinessObjects’ Data Integrator - BODI), SAS, Ab Initio, And Talend Lead, With Pervasive, Microsoft (SSIS), And iWay Close Behind in The Forrester Wave™ Enterprise ETL, Q1 2012.
Real-Time, Near Real-Time & Right-Time Business Intelligence
It is important for Enterprise to have the data, reports, alerts, predictions and information at right time rather struggling for Real Time & Near Real time data acquisitions and integration. Everyone is boasting about the Real Time and near real time, but what is the realization? Are we using the data at right time?
Real-time business intelligence (RTBI) is the process of delivering information about business operations as they occur.
The speed of today's processing systems has moved classical data warehousing into the realm of real-time. The result is real-time business intelligence. Business transactions as they occur are fed to a real-time business intelligence system that maintains the current state of the enterprise. The RTBI system not only supports the classic strategic functions of data warehousing for deriving information and knowledge from past enterprise activity, but it also provides real-time tactical support to drive enterprise actions that react immediately to events as they occur. As such, it replaces both the classic data warehouse and the enterprise application integration (EAI) functions. Such event-driven processing is a basic tenet of real-time business intelligence
All real-time business intelligence systems have some latency, but the goal is to minimize the time from the business event happening to a corrective action or notification being initiated. Analyst Richard Hackathorn describes three types of latency:
1. Data latency; the time taken to collect and store the data
2. Analysis latency; the time taken to analyze the data and turn it into actionable information
3. Action latency; the time taken to react to the information and take action
Real-time business intelligence technologies are designed to reduce all three latencies to as close to zero as possible, whereas traditional business intelligence only seeks to reduce data latency and does not address analysis latency or action latency since both are governed by manual processes.
The term "near real-time" or "nearly real-time" (NRT), in telecommunications and computing, refers to the time delay introduced, by automated data processing or network transmission, between the occurrence of an event and the use of the processed data, such as for display or feedback and control purposes. For example, a near-real-time display depicts an event or situation as it existed at the current time minus the processing time, as nearly the time of the live event.
The distinction between the terms "near real time" and "real time" is somewhat nebulous and must be defined for the situation at hand. The term implies that there are no significant delays. In many cases, processing described as "real-time" would be more accurately described as "near-real-time".
The Change Data Capture (CDC) tool is booming to address the Real-Time, Near Real-Time data integration. Now the data is growing rapidly and the address the business ineed we must understand the need of Right-Time data and information.
Real-time business intelligence (RTBI) is the process of delivering information about business operations as they occur.
The speed of today's processing systems has moved classical data warehousing into the realm of real-time. The result is real-time business intelligence. Business transactions as they occur are fed to a real-time business intelligence system that maintains the current state of the enterprise. The RTBI system not only supports the classic strategic functions of data warehousing for deriving information and knowledge from past enterprise activity, but it also provides real-time tactical support to drive enterprise actions that react immediately to events as they occur. As such, it replaces both the classic data warehouse and the enterprise application integration (EAI) functions. Such event-driven processing is a basic tenet of real-time business intelligence
All real-time business intelligence systems have some latency, but the goal is to minimize the time from the business event happening to a corrective action or notification being initiated. Analyst Richard Hackathorn describes three types of latency:
1. Data latency; the time taken to collect and store the data
2. Analysis latency; the time taken to analyze the data and turn it into actionable information
3. Action latency; the time taken to react to the information and take action
Real-time business intelligence technologies are designed to reduce all three latencies to as close to zero as possible, whereas traditional business intelligence only seeks to reduce data latency and does not address analysis latency or action latency since both are governed by manual processes.
The term "near real-time" or "nearly real-time" (NRT), in telecommunications and computing, refers to the time delay introduced, by automated data processing or network transmission, between the occurrence of an event and the use of the processed data, such as for display or feedback and control purposes. For example, a near-real-time display depicts an event or situation as it existed at the current time minus the processing time, as nearly the time of the live event.
The distinction between the terms "near real time" and "real time" is somewhat nebulous and must be defined for the situation at hand. The term implies that there are no significant delays. In many cases, processing described as "real-time" would be more accurately described as "near-real-time".
The Change Data Capture (CDC) tool is booming to address the Real-Time, Near Real-Time data integration. Now the data is growing rapidly and the address the business ineed we must understand the need of Right-Time data and information.
Monday, February 27, 2012
Ten Rules for Next Generation Data Integration (By philip russom, TDWI)
I was going through Ten Rules for Next Generation Data Integration (By philip russom, TDWI) and found really interesting. Follows the jist of the deck:
Data integration (DI) has undergone an impressive evolution in recent years. Today, DI is a rich set of powerful techniques, including ETL (extract, transform, and load), data federation, replication, synchronization, changed data capture, data quality, master data management, natural language processing, business-to business data exchange, and more. Furthermore, vendor products for DI have achieved maturity, users have grown their DI teams to epic proportions, competency centers regularly staff DI work, new best practices continue to arise (such as collaborative DI and agile DI), and DI as a discipline has earned its autonomy from related practices such as data warehousing and database administration.
Ten Rules for Next Generation Data Integration
1. DI is a family of techniques.
2. DI techniques may be hand coded, based on a vendor’s tool, or both.
3. DI practices reach across both analytics and operations.
4. DI is an autonomous discipline.
5. DI is absorbing other data management disciplines.
6. DI has become broadly collaborative.
7. DI needs diverse development methodologies.
8. DI requires a wide range of interfaces.
9. DI must scale.
10. DI requires architecture.
Why Care About next generation data integration (NGDI) Now?
Businesses face change more often than ever before.
Even mature DI solutions have room to grow.
The next generation is an opportunity to fix the failings of prior generations.
For many, the next generation is about tapping more functions of DI tools they already have.
Unstructured data is still an unexplored frontier for most DI solutions.
DI is on its way to becoming IT infrastructure.DI is a growing and evolving practice.
Data integration (DI) has undergone an impressive evolution in recent years. Today, DI is a rich set of powerful techniques, including ETL (extract, transform, and load), data federation, replication, synchronization, changed data capture, data quality, master data management, natural language processing, business-to business data exchange, and more. Furthermore, vendor products for DI have achieved maturity, users have grown their DI teams to epic proportions, competency centers regularly staff DI work, new best practices continue to arise (such as collaborative DI and agile DI), and DI as a discipline has earned its autonomy from related practices such as data warehousing and database administration.
Ten Rules for Next Generation Data Integration
1. DI is a family of techniques.
2. DI techniques may be hand coded, based on a vendor’s tool, or both.
3. DI practices reach across both analytics and operations.
4. DI is an autonomous discipline.
5. DI is absorbing other data management disciplines.
6. DI has become broadly collaborative.
7. DI needs diverse development methodologies.
8. DI requires a wide range of interfaces.
9. DI must scale.
10. DI requires architecture.
Why Care About next generation data integration (NGDI) Now?
Businesses face change more often than ever before.
Even mature DI solutions have room to grow.
The next generation is an opportunity to fix the failings of prior generations.
For many, the next generation is about tapping more functions of DI tools they already have.
Unstructured data is still an unexplored frontier for most DI solutions.
DI is on its way to becoming IT infrastructure.DI is a growing and evolving practice.
Friday, February 24, 2012
The New Analytical Ecosystem: Making Way for Big Data (By Wayne Eckerson)
I was going through Wayne Eckerson blog and find really interesting about this article.
The top-down world. In the top-down world, source data is processed, refined, and stamped with a predefined data structure--typically a dimensional model--and then consumed by casual users using SQL-based reporting and analysis tools. In this domain, IT developers create data and semantic models so business users can get answers to known questions and executives can track performance of predefined metrics. Here, design precedes access. The top-down world also takes great pains to align data along conformed dimensions and deliver clean, accurate data. The goal is to deliver a consistent view of the business entities so users can spend their time making decisions instead of arguing about the origins and validity of data artifacts.
The under world. Creating a uniform view of the business from heterogeneous sets of data is not easy. It takes time, money, and patience, often more than most departmental heads and business analysts are willing to tolerate. They often abandon the top-down world for the underworld of spreadmarts and data shadow systems. Using whatever tools are readily available and cheap, these data hungry users create their own views of the business. Eventually, they spend more time collecting and integrating data than analyzing it, undermining their productivity and a consistent view of business information.
The bottom up world. The new analytical ecosystem brings these prodigal data users back into the fold. It carves out space within the enterprise environment for true ad hoc exploration and promotes the rapid development of analytical applications using in-memory departmental tools. In a bottom-up environment, users can't anticipate the questions they will ask on a daily or weekly basis or the data they'll need to answer those questions. Often, the data they need doesn't yet exist in the data warehouse.
The new analytical ecosystem creates analytical sandboxes that let power users explore corporate and local data on their own terms. These sandboxes include Hadoop, virtual partitions inside a data warehouse, and specialized analytical databases that offload data or analytical processing from the data warehouse or handle new untapped sources of data, such as Web logs or machine data. The new environment also gives department heads the ability to create and consume dashboards built with in-memory visualization tools that point both to a corporate data warehouse and other independent sources.
Combining top-down and bottom-up worlds is not easy. BI professionals need to assiduously guard data semantics while opening access to data. For their part, business users need to commit to adhering to corporate data standards in exchange for getting the keys to the kingdom. To succeed, organizations need robust data governance programs and lots of communication among all parties.
Summary. The Big Data revolution brings major enhancements to the BI landscape. First and foremost, it introduces new technologies, such as Hadoop, that make it possible for organizations to cost-effectively consume and analyze large volumes of semi-structured data. Second, it complements traditional top-down data delivery methods with more flexible, bottom-up approaches that promote ad hoc exploration and rapid application development.
The top-down world. In the top-down world, source data is processed, refined, and stamped with a predefined data structure--typically a dimensional model--and then consumed by casual users using SQL-based reporting and analysis tools. In this domain, IT developers create data and semantic models so business users can get answers to known questions and executives can track performance of predefined metrics. Here, design precedes access. The top-down world also takes great pains to align data along conformed dimensions and deliver clean, accurate data. The goal is to deliver a consistent view of the business entities so users can spend their time making decisions instead of arguing about the origins and validity of data artifacts.
The under world. Creating a uniform view of the business from heterogeneous sets of data is not easy. It takes time, money, and patience, often more than most departmental heads and business analysts are willing to tolerate. They often abandon the top-down world for the underworld of spreadmarts and data shadow systems. Using whatever tools are readily available and cheap, these data hungry users create their own views of the business. Eventually, they spend more time collecting and integrating data than analyzing it, undermining their productivity and a consistent view of business information.
The bottom up world. The new analytical ecosystem brings these prodigal data users back into the fold. It carves out space within the enterprise environment for true ad hoc exploration and promotes the rapid development of analytical applications using in-memory departmental tools. In a bottom-up environment, users can't anticipate the questions they will ask on a daily or weekly basis or the data they'll need to answer those questions. Often, the data they need doesn't yet exist in the data warehouse.
The new analytical ecosystem creates analytical sandboxes that let power users explore corporate and local data on their own terms. These sandboxes include Hadoop, virtual partitions inside a data warehouse, and specialized analytical databases that offload data or analytical processing from the data warehouse or handle new untapped sources of data, such as Web logs or machine data. The new environment also gives department heads the ability to create and consume dashboards built with in-memory visualization tools that point both to a corporate data warehouse and other independent sources.
Combining top-down and bottom-up worlds is not easy. BI professionals need to assiduously guard data semantics while opening access to data. For their part, business users need to commit to adhering to corporate data standards in exchange for getting the keys to the kingdom. To succeed, organizations need robust data governance programs and lots of communication among all parties.
Summary. The Big Data revolution brings major enhancements to the BI landscape. First and foremost, it introduces new technologies, such as Hadoop, that make it possible for organizations to cost-effectively consume and analyze large volumes of semi-structured data. Second, it complements traditional top-down data delivery methods with more flexible, bottom-up approaches that promote ad hoc exploration and rapid application development.
Friday, February 17, 2012
Gartner's Magic Quadrant for Business Intelligence Platform 2012
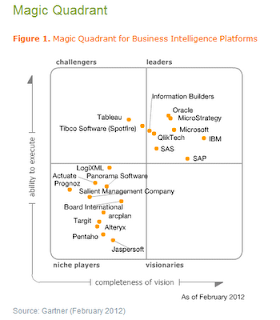
IBM-Cognos, Microsoft-SSAS, Oracle-OBIEE, SAP-Business Object, Microstrategy, Information Builder, QlikTech & SAS are in the Leader's quadrant in 2012 Magic Quadrant for BI Platforms . Tableau & Tibco-Spotfire are in the challengers quadrant and few new entrants (ALTERYX. PROGNOZ).
For more please refer: http://www.gartner.com/technology/reprints.do?id=1-196WFFX&ct=120207&st=sb
For more please refer: http://www.gartner.com/technology/reprints.do?id=1-196WFFX&ct=120207&st=sb
Sunday, February 5, 2012
The Forrester Wave™: Enterprise Hadoop Solutions, Q1 2012
Wednesday, February 1, 2012
From Business Intelligence to Intelligent Business (By Gartner)
Intelligence cannot be bought. An intelligent business develops the right culture and processes to ensure that the right information is available for people to make the right decisions at all levels of the organization.
Key Findings
# Information required for effective decisions comes from many diverse sources.
# Too much information can be as bad as too little information.
# Intelligent business develops by linking process and information integration with business strategies.
Recommendations
# Focus business intelligence (BI) efforts on delivering the right information to the right people at the right time to impact critical business decisions in key business processes.
# Change the mind-set from one that simply demands more information to one in which asking the right questions drives impactful decisions.
# Create project teams based not on data ownership but on information needs up and down the management chains and across functions to drive maximum decision impact.
Analysis
Check the websites of ERP, BI and content management vendors, and a common thread is "buy our product and receive best-practice solutions." If it were as simple as this, most of the major enterprises around the globe would be at world's best practice, because they have generally invested in data warehouses and BI tools that have become progressively more mature.
But ask a range of business leaders "Does everyone in your organization have the right information in the right format at the right time to make the best possible decisions?" and the common answer is "No." One reason is that the information required to make many decisions does not reside in any convenient databases but comes from a complex mix of sources, including e-mails, voice messages, personal experience, multimedia information, and external communications from suppliers, customers, governments and so on.
Traditionally, BI has been used for performance reporting from historical data and as a planning and forecasting tool for a relatively small subset of those in the organization who rely on historical data to create a crystal ball for looking into the future.
Providing real-time information derived from a fusion of data and analytical tools with key business applications such as call center, CRM or ERP creates the ability to push alerts to knowledge or process workers and represents a significant effectiveness impact on decision making, driving additional revenue, margin or client satisfaction. Modeling future scenarios permits examination of new business models, new market opportunities and new products, and creates a culture of "Which opportunities will we seize?" They not only see the future, but often create it.
Case studies presented in Executive Program reports highlight initiatives that are exploiting business intelligence to create intelligent businesses. Three key recommendations are:
1. Focus BI efforts on delivering the right information to the right people.
2. Change the mind-set from more information to answering the right questions.
3. Create project teams based on information needs.
Key Findings
# Information required for effective decisions comes from many diverse sources.
# Too much information can be as bad as too little information.
# Intelligent business develops by linking process and information integration with business strategies.
Recommendations
# Focus business intelligence (BI) efforts on delivering the right information to the right people at the right time to impact critical business decisions in key business processes.
# Change the mind-set from one that simply demands more information to one in which asking the right questions drives impactful decisions.
# Create project teams based not on data ownership but on information needs up and down the management chains and across functions to drive maximum decision impact.
Analysis
Check the websites of ERP, BI and content management vendors, and a common thread is "buy our product and receive best-practice solutions." If it were as simple as this, most of the major enterprises around the globe would be at world's best practice, because they have generally invested in data warehouses and BI tools that have become progressively more mature.
But ask a range of business leaders "Does everyone in your organization have the right information in the right format at the right time to make the best possible decisions?" and the common answer is "No." One reason is that the information required to make many decisions does not reside in any convenient databases but comes from a complex mix of sources, including e-mails, voice messages, personal experience, multimedia information, and external communications from suppliers, customers, governments and so on.
Traditionally, BI has been used for performance reporting from historical data and as a planning and forecasting tool for a relatively small subset of those in the organization who rely on historical data to create a crystal ball for looking into the future.
Providing real-time information derived from a fusion of data and analytical tools with key business applications such as call center, CRM or ERP creates the ability to push alerts to knowledge or process workers and represents a significant effectiveness impact on decision making, driving additional revenue, margin or client satisfaction. Modeling future scenarios permits examination of new business models, new market opportunities and new products, and creates a culture of "Which opportunities will we seize?" They not only see the future, but often create it.
Case studies presented in Executive Program reports highlight initiatives that are exploiting business intelligence to create intelligent businesses. Three key recommendations are:
1. Focus BI efforts on delivering the right information to the right people.
2. Change the mind-set from more information to answering the right questions.
3. Create project teams based on information needs.
Thursday, January 26, 2012
Row-Based Vs Columnar Vs NoSQL
There are various Database players in the market. Here is one quick comparsion on Row-Based Vs Vs Columnar Vs NoSQL.
Row-based
Description: Data structured or stored in Rows.
Common Use Case: Used in transaction processing, interactive transaction applications.
Strength: Robust, proven technology to capture intermediate transactions.
Weakness: Scalability and query processing time for huge data.
Size of DB: Several GB to TB.
Key Players: Sybase, Oracle, My SQL, DB2
Columnar
Description: Data is vertically partitioned and stored in Columns.
Common Use Case: Historical data analysis, data warehousing and business Intelligence.
Strength: Faster query (specially ad-hoc queries) on large data.
Weakness: not suitable for transaction, import export seep & heavy computing resource utilization.
Size of DB: Several GB to 50 TB.
Key Players: Info Bright, Asterdata, Vertica, Sybase IQ, Paraccel
NoSQL Key Value Stored
Description: Data stored in memory with some persistent backup.
Common Use Case: Used in cache for storing frequently requested data in applications.
Strength: Scalable, faster retrieval of data , supports Unstructured and partial structured data.
Weakness: All data should fit to memory, does not support complex query.
Size of DB: Several GBs to several TBs.
Key Players: Amazon S3, MemCached, Redis, Voldemort
NoSQL- Document Store
Description: Persistent storage of unstructured or semi-structured data along with some SQL Querying functionality.
Common Use Case: Web applications or any application which needs better performance and scalability without defining columns in RDBMS.
Strength: Persistent store with scalability and better query support than key-value store.
Weakness: Lack of sophisticated query capabilities.
Size of DB: Several TBs to PBs.
Key Players: MongoDB, CouchDB, SimpleDb
NoSQL- Column Store
Description: Very large data store and supports Map-Reduce.
Common Use Case: Real time data logging in Finance and web analytics.
Strength: Very high throughput for Big Data, Strong Partitioning Support, random read-write access.
Weakness: Complex query, availability of APIs, response time.
Size of DB: Several TBs to PBs.Key Players: HBase, Big Table, Cassandra
Row-based
Description: Data structured or stored in Rows.
Common Use Case: Used in transaction processing, interactive transaction applications.
Strength: Robust, proven technology to capture intermediate transactions.
Weakness: Scalability and query processing time for huge data.
Size of DB: Several GB to TB.
Key Players: Sybase, Oracle, My SQL, DB2
Columnar
Description: Data is vertically partitioned and stored in Columns.
Common Use Case: Historical data analysis, data warehousing and business Intelligence.
Strength: Faster query (specially ad-hoc queries) on large data.
Weakness: not suitable for transaction, import export seep & heavy computing resource utilization.
Size of DB: Several GB to 50 TB.
Key Players: Info Bright, Asterdata, Vertica, Sybase IQ, Paraccel
NoSQL Key Value Stored
Description: Data stored in memory with some persistent backup.
Common Use Case: Used in cache for storing frequently requested data in applications.
Strength: Scalable, faster retrieval of data , supports Unstructured and partial structured data.
Weakness: All data should fit to memory, does not support complex query.
Size of DB: Several GBs to several TBs.
Key Players: Amazon S3, MemCached, Redis, Voldemort
NoSQL- Document Store
Description: Persistent storage of unstructured or semi-structured data along with some SQL Querying functionality.
Common Use Case: Web applications or any application which needs better performance and scalability without defining columns in RDBMS.
Strength: Persistent store with scalability and better query support than key-value store.
Weakness: Lack of sophisticated query capabilities.
Size of DB: Several TBs to PBs.
Key Players: MongoDB, CouchDB, SimpleDb
NoSQL- Column Store
Description: Very large data store and supports Map-Reduce.
Common Use Case: Real time data logging in Finance and web analytics.
Strength: Very high throughput for Big Data, Strong Partitioning Support, random read-write access.
Weakness: Complex query, availability of APIs, response time.
Size of DB: Several TBs to PBs.Key Players: HBase, Big Table, Cassandra
Wednesday, January 18, 2012
Cloud BI The reality (From Warne's blog)
Three Types of Cloud Services with BI Examples
Software-as-a-Service (SaaS). SaaS delivers packaged applications tailored to specific workflows and users. SaaS was first popularized by Salesforce.com, which was founded in 1996 to deliver online sales applications to small- and medium-sized businesses. Salesforce.com now has 92,000 customers of all sizes and has spawned a multitude of imitators. A big benefit of SaaS is that it obviates the need for customers to maintain and upgrade application code and infrastructure. Many SaaS customers are astonished to see new software features automatically appear in their application without notice or additional expense.
Within the BI market, many startups and established BI players offer SaaS BI services that deliver ready-made reports and dashboards for specific commercial applications, such as Salesforce, NetSuite, Microsoft Dynamics, and others. SaaS BI vendors include Birst, PivotLink, GoodData, Indicee, Rosslyn Analytics, and SAP, among others.
Platform-as-a-Service (PaaS). PaaS enables developers to build applications online. PaaS services provide development environments, such as programming languages and databases, so developers can create and deliver applications without having to purchase and install hardware. In the BI market, the SaaS BI vendors (above) for the most part double as PaaS BI vendors.
In a PaaS environment, a developer must first build a data mart, which is often tedious and highly customized work since it involves integrating data from multiple sources, cleaning and standardizing the data, and finally modeling and transforming the data. Although SaaS BI applications deploy quickly, PaaS BI applications are not. Basically, SaaS BI are packaged applications while PaaS BI are custom applications. In the world of BI, most applications are custom. This is the primary reason why growth of Cloud BI in general is slower than anticipated.
SaaS BI are packaged applications and PaaS BI are custom applications. In the world of BI, most applications are custom.
Infrastructure-as-a-Service (IaaS). IaaS provides online computing resources (servers, storage, and networking) which customers use to augment or replace their existing compute resources. In 2006, Amazon popularized IaaS when it began renting space in its own data center using virtualization services to outside parties. Some BI vendors are beginning to offer software infrastructure within public cloud or hosted environments. For example, analytic databases Vertica and Teradata are now available as public services within Amazon EC2, while Kognitio offers a private hosted service. ETL vendors Informatica and SnapLogic also offer services in the cloud.
Key Characteristics of the Cloud
Virtualization is the foundation of cloud computing. You can’t do cloud computing without virtualization; but virtualization by itself doesn’t constitute cloud computing.
Virtualization abstracts or virtualizes the underlying compute infrastructure using a piece of software called a hypervisor. With virtualization, you create virtual servers (or virtual machines) to run your applications. Your virtual server can have a different operating system than the physical hardware upon which it is running. For the most part, users no longer have to worry whether they have the right operating system, hardware, and networking to support a BI or other application. Virtualization shields users and developers from the underlying complexity of the compute infrastructure (as long as the IT department has created appropriate virtual machines for them to use.)
Deployment Options for Cloud Computing
Public Cloud. Application and compute resources are managed by a third party services provider.
Private Cloud. Application and compute resources are managed by an internal data center team.
Hybrid Cloud. Either a private cloud that leverages the public cloud to handle peak capacity, or a reserved “private” space within a public cloud, or a hybrid architecture in which some components run in a data center and others in the public cloud.
Software-as-a-Service (SaaS). SaaS delivers packaged applications tailored to specific workflows and users. SaaS was first popularized by Salesforce.com, which was founded in 1996 to deliver online sales applications to small- and medium-sized businesses. Salesforce.com now has 92,000 customers of all sizes and has spawned a multitude of imitators. A big benefit of SaaS is that it obviates the need for customers to maintain and upgrade application code and infrastructure. Many SaaS customers are astonished to see new software features automatically appear in their application without notice or additional expense.
Within the BI market, many startups and established BI players offer SaaS BI services that deliver ready-made reports and dashboards for specific commercial applications, such as Salesforce, NetSuite, Microsoft Dynamics, and others. SaaS BI vendors include Birst, PivotLink, GoodData, Indicee, Rosslyn Analytics, and SAP, among others.
Platform-as-a-Service (PaaS). PaaS enables developers to build applications online. PaaS services provide development environments, such as programming languages and databases, so developers can create and deliver applications without having to purchase and install hardware. In the BI market, the SaaS BI vendors (above) for the most part double as PaaS BI vendors.
In a PaaS environment, a developer must first build a data mart, which is often tedious and highly customized work since it involves integrating data from multiple sources, cleaning and standardizing the data, and finally modeling and transforming the data. Although SaaS BI applications deploy quickly, PaaS BI applications are not. Basically, SaaS BI are packaged applications while PaaS BI are custom applications. In the world of BI, most applications are custom. This is the primary reason why growth of Cloud BI in general is slower than anticipated.
SaaS BI are packaged applications and PaaS BI are custom applications. In the world of BI, most applications are custom.
Infrastructure-as-a-Service (IaaS). IaaS provides online computing resources (servers, storage, and networking) which customers use to augment or replace their existing compute resources. In 2006, Amazon popularized IaaS when it began renting space in its own data center using virtualization services to outside parties. Some BI vendors are beginning to offer software infrastructure within public cloud or hosted environments. For example, analytic databases Vertica and Teradata are now available as public services within Amazon EC2, while Kognitio offers a private hosted service. ETL vendors Informatica and SnapLogic also offer services in the cloud.
Key Characteristics of the Cloud
Virtualization is the foundation of cloud computing. You can’t do cloud computing without virtualization; but virtualization by itself doesn’t constitute cloud computing.
Virtualization abstracts or virtualizes the underlying compute infrastructure using a piece of software called a hypervisor. With virtualization, you create virtual servers (or virtual machines) to run your applications. Your virtual server can have a different operating system than the physical hardware upon which it is running. For the most part, users no longer have to worry whether they have the right operating system, hardware, and networking to support a BI or other application. Virtualization shields users and developers from the underlying complexity of the compute infrastructure (as long as the IT department has created appropriate virtual machines for them to use.)
Deployment Options for Cloud Computing
Public Cloud. Application and compute resources are managed by a third party services provider.
Private Cloud. Application and compute resources are managed by an internal data center team.
Hybrid Cloud. Either a private cloud that leverages the public cloud to handle peak capacity, or a reserved “private” space within a public cloud, or a hybrid architecture in which some components run in a data center and others in the public cloud.
Monday, January 9, 2012
Types of Analytical Platforms
MPP analytical databases : Row-based databases designed to scale out on a cluster of commodity servers and run complex queries in parallel against large volumes of data.
[Teradata Active Data Warehouse, Greenplum (EMC), Microsoft Parallel Data Warehouse, Aster Data (Teradata), Kognitio, Dataupia]
Columnar databases : Database management systems that store data in columns, not rows, and support high data compression ratios.
[ParAccel, Infobright, Sand Technology, Sybase IQ (SAP), Vertica (Hewlett-Packard), 1010data, Exasol, Calpont]
Analytical appliances : Preconfigured hardware-software systems designed for query processing and analytics that require little tuning.
[Netezza (IBM), Teradata Appliances, Oracle Exadata, Greenplum Data Computing Appliance (EMC)]
Analytical bundles : Predefined hardware and software configurations that are certified to meet specific performance criteria, but the customer must purchase and configure themselves.
[IBM SmartAnalytics, Microsoft FastTrack]
In-memory databases : Systems that load data into memory to execute complex queries.
[SAP HANA, Cognos TM1 (IBM), QlikView, Membase]
Distributed file-based systems : Distributed file systems designed for storing, indexing, manipulating and querying large volumes of unstructured and semi-structured data.
[Hadoop (Apache, Cloudera, MapR, IBM, HortonWorks), Apache Hive, Apache Pig]
Analytical services : Analytical platforms delivered as a hosted or public-cloud-based service.
[1010data, Kognitio]
Nonrelational : Nonrelational databases optimized for querying unstructured data as well as structured data.
[MarkLogic Server, MongoDB, Splunk, Attivio, Endeca, Apache Cassandra, Apache Hbase]
CEP/streaming engines: Ingest, filter, calculate, and correlate large volumes of discrete events and apply rules that trigger alerts when conditions are met.
[IBM, Tibco, Streambase, Sybase (Aleri), Opalma, Vitria, Informatica]
[Teradata Active Data Warehouse, Greenplum (EMC), Microsoft Parallel Data Warehouse, Aster Data (Teradata), Kognitio, Dataupia]
Columnar databases : Database management systems that store data in columns, not rows, and support high data compression ratios.
[ParAccel, Infobright, Sand Technology, Sybase IQ (SAP), Vertica (Hewlett-Packard), 1010data, Exasol, Calpont]
Analytical appliances : Preconfigured hardware-software systems designed for query processing and analytics that require little tuning.
[Netezza (IBM), Teradata Appliances, Oracle Exadata, Greenplum Data Computing Appliance (EMC)]
Analytical bundles : Predefined hardware and software configurations that are certified to meet specific performance criteria, but the customer must purchase and configure themselves.
[IBM SmartAnalytics, Microsoft FastTrack]
In-memory databases : Systems that load data into memory to execute complex queries.
[SAP HANA, Cognos TM1 (IBM), QlikView, Membase]
Distributed file-based systems : Distributed file systems designed for storing, indexing, manipulating and querying large volumes of unstructured and semi-structured data.
[Hadoop (Apache, Cloudera, MapR, IBM, HortonWorks), Apache Hive, Apache Pig]
Analytical services : Analytical platforms delivered as a hosted or public-cloud-based service.
[1010data, Kognitio]
Nonrelational : Nonrelational databases optimized for querying unstructured data as well as structured data.
[MarkLogic Server, MongoDB, Splunk, Attivio, Endeca, Apache Cassandra, Apache Hbase]
CEP/streaming engines: Ingest, filter, calculate, and correlate large volumes of discrete events and apply rules that trigger alerts when conditions are met.
[IBM, Tibco, Streambase, Sybase (Aleri), Opalma, Vitria, Informatica]
Why many BI implementations across organizations fails?
# Failure to tie the Business Intelligence Strategy with the Enterprise vision & Strategy.
# Failure to have a flexible BI Architecture that aligns with the Enterprise I.T Architecture.
# Failure to have Enterprise vision of Data Quality, Master Data Management, Metadata Management, Portalization ..etc
# Business Adoptability.
# Various BI tools seamless integration.
# Over-all Business pattern as well as technology is changing. (Example, envisioning DW 2.0 as well as the Business need while architecting)
# Calculating the ROI for any BI implementation and realization takes little longer as compared to other programs.
# Failure due to projects being driven by I.T instead of the business.
# Failure due to business not being involved heavily right from the beginning.
# Lack of good understanding of the BI and Data Warehousing fundamentals and concepts.
# Failure by deviating from the objective and focus during the phases of BI project.
# Lack of BI skills in team implementation team.
# Failure to have a clear working process between the Infrastructures, ETL & Reporting team.
# Lack of continuous support & direction from the leadership team.
# Lack of a clear and detailed BI strategy and road map.
# Failure to gather complete requirements and do system study.
# Lack of a clear understanding of the end goal.
# Lack of a an end to end understanding of the initiative with the source systems team, Infrastructure team, ETL team, Reporting & Analytics team and the Business all functioning in silos.
# Lack of continuous training for the implementation team and the business.
# Lack of a clear support structure.
# Lack of enough budgets that delays upgrades and feature enhancements.
# Implementation of BI plans, direction and work structure by a PMO or Business team without the involvement or consultation of the BI team.
# Lack of clear testing by the UAT team.
# Incorrect and non-flexible data model.
# Lack of clear communication and co-ordination issues between different teams and to the business stake holders.
# Lack of a proper change management process.
# Failure to have a scope creep and breaking the deliverable into phases as there is never an end to the requirements sometimes as BI is iterative and business expects constant delivery of values.
# Failure to include performance criteria as part of the BI and Analytics deliverable.
# Failure to determine and include SLA's for each piece of the BI.
# Failure to have a flexible BI Architecture that aligns with the Enterprise I.T Architecture.
# Failure to have Enterprise vision of Data Quality, Master Data Management, Metadata Management, Portalization ..etc
# Business Adoptability.
# Various BI tools seamless integration.
# Over-all Business pattern as well as technology is changing. (Example, envisioning DW 2.0 as well as the Business need while architecting)
# Calculating the ROI for any BI implementation and realization takes little longer as compared to other programs.
# Failure due to projects being driven by I.T instead of the business.
# Failure due to business not being involved heavily right from the beginning.
# Lack of good understanding of the BI and Data Warehousing fundamentals and concepts.
# Failure by deviating from the objective and focus during the phases of BI project.
# Lack of BI skills in team implementation team.
# Failure to have a clear working process between the Infrastructures, ETL & Reporting team.
# Lack of continuous support & direction from the leadership team.
# Lack of a clear and detailed BI strategy and road map.
# Failure to gather complete requirements and do system study.
# Lack of a clear understanding of the end goal.
# Lack of a an end to end understanding of the initiative with the source systems team, Infrastructure team, ETL team, Reporting & Analytics team and the Business all functioning in silos.
# Lack of continuous training for the implementation team and the business.
# Lack of a clear support structure.
# Lack of enough budgets that delays upgrades and feature enhancements.
# Implementation of BI plans, direction and work structure by a PMO or Business team without the involvement or consultation of the BI team.
# Lack of clear testing by the UAT team.
# Incorrect and non-flexible data model.
# Lack of clear communication and co-ordination issues between different teams and to the business stake holders.
# Lack of a proper change management process.
# Failure to have a scope creep and breaking the deliverable into phases as there is never an end to the requirements sometimes as BI is iterative and business expects constant delivery of values.
# Failure to include performance criteria as part of the BI and Analytics deliverable.
# Failure to determine and include SLA's for each piece of the BI.
Thursday, January 5, 2012
Why Big Data? (By WAYNE ECKERSON)
Why Big Data? (By WAYNE ECKERSON)
There has been a lot of talk about “big data” in the past year, which I find a bit puzzling. I’ve been in the data warehousing field for more than 15 years, and data warehousing has always been about big data. So what’s new in 2011? Why are we are talking about “big data” today? There are several reasons:
Changing data types. Organizations are capturing different types of data today. Until about five years ago, most data was transactional in nature, consisting of numeric data that fit easily into rows and columns of relational databases. Today, the growth in data is fueled by largely unstructured data from wWeb sites as well as machine-generated data from an exploding number of sensors.
Technology advances. Hardware has finally caught up with software. The exponential gains in price/-performance exhibited by computer processors, memory, and disk storage have finally made it possible to store and analyze large volumes of data at an affordable price. Organizations are storing and analyzing more data because they can.!
Insourcing and outsourcing. Because of the complexity and cost of storing and analyzing Web traffic data, most organizations have outsourced these functions to third- party service bureaus. But as the size and importance of corporate e-commerce channels have increased, many are now eager to insource this data to gain greater insights about customers. At the same time, virtualization technology is making it attractive for organizations to move large-scale data processing to private hosted networks or public clouds.
Developers discover data. The biggest reason for the popularity of the term “big data” is that Web and application developers have discovered the value of building a new data-intensive applications. To application developers, “big data” is new and exciting. Of course, for those of us who have made their careers in the data world, the new era of “big data” is simply another step in the evolution of data management systems that support reporting and analysis applications.
Analytics against Big Data
Big data by itself, regardless of the type, is worthless unless business users do something with it that delivers value to their organizations. That’s where analytics comes in. Although organizations have always run reports against data warehouses, most haven’t opened these repositories to ad hoc exploration. This is partly because analysis tools are too complex for the average user but also because the repositories often don’t contain all the data needed by the power user. But this is changing.
• Patterns. A valuable characteristic of ““big data”” is that it contains more patterns and interesting anomalies than “small” data. Thus, organizations can gain greater value by mining large data volumes than small ones. Fortunately, techniques already exist to mine big data thanks to companies, such as SAS Institute and SPSS (now part of IBM), that ship analytical workbenches.
• Real-time. Organizations that accumulate big data recognize quickly that they need to change the way they capture, transform, and move data from a nightly batch process to a continuous process using micro batch loads or event-driven updates. This technical constraint pays big business dividends because it makes it possible to deliver critical information to users in near- real- time.
• Complex analytics. In addition, during the past 15 years, the “analytical IQ” of many organizations has evolved from reporting and dashboarding to lightweight analysis. Many are now on the verge of upping their analytical IQ by implementing predictive analytics against both structured and unstructured data. This type of analytics can be used to do everything from delivered highly tailored cross-sell recommendations to predicting failure rates of aircraft engines.
• Sustainable advantage . At the same time, executives have recognized the power of analytics to deliver a competitive advantage, thanks to the pioneering work of thought leaders, such as Tom Davenport, who co-wrote the book, “Competing on Analytics.” In fact, forward-thinking executives recognize that analytics may be the only true source of sustainable advantage since it empowers employees at all levels of an organization with information to help them make smarter decisions.
For more refer: http://docs.media.bitpipe.com/io_10x/io_101195/item_472252/Research%20report%20Chapter%201update.pdf
There has been a lot of talk about “big data” in the past year, which I find a bit puzzling. I’ve been in the data warehousing field for more than 15 years, and data warehousing has always been about big data. So what’s new in 2011? Why are we are talking about “big data” today? There are several reasons:
Changing data types. Organizations are capturing different types of data today. Until about five years ago, most data was transactional in nature, consisting of numeric data that fit easily into rows and columns of relational databases. Today, the growth in data is fueled by largely unstructured data from wWeb sites as well as machine-generated data from an exploding number of sensors.
Technology advances. Hardware has finally caught up with software. The exponential gains in price/-performance exhibited by computer processors, memory, and disk storage have finally made it possible to store and analyze large volumes of data at an affordable price. Organizations are storing and analyzing more data because they can.!
Insourcing and outsourcing. Because of the complexity and cost of storing and analyzing Web traffic data, most organizations have outsourced these functions to third- party service bureaus. But as the size and importance of corporate e-commerce channels have increased, many are now eager to insource this data to gain greater insights about customers. At the same time, virtualization technology is making it attractive for organizations to move large-scale data processing to private hosted networks or public clouds.
Developers discover data. The biggest reason for the popularity of the term “big data” is that Web and application developers have discovered the value of building a new data-intensive applications. To application developers, “big data” is new and exciting. Of course, for those of us who have made their careers in the data world, the new era of “big data” is simply another step in the evolution of data management systems that support reporting and analysis applications.
Analytics against Big Data
Big data by itself, regardless of the type, is worthless unless business users do something with it that delivers value to their organizations. That’s where analytics comes in. Although organizations have always run reports against data warehouses, most haven’t opened these repositories to ad hoc exploration. This is partly because analysis tools are too complex for the average user but also because the repositories often don’t contain all the data needed by the power user. But this is changing.
• Patterns. A valuable characteristic of ““big data”” is that it contains more patterns and interesting anomalies than “small” data. Thus, organizations can gain greater value by mining large data volumes than small ones. Fortunately, techniques already exist to mine big data thanks to companies, such as SAS Institute and SPSS (now part of IBM), that ship analytical workbenches.
• Real-time. Organizations that accumulate big data recognize quickly that they need to change the way they capture, transform, and move data from a nightly batch process to a continuous process using micro batch loads or event-driven updates. This technical constraint pays big business dividends because it makes it possible to deliver critical information to users in near- real- time.
• Complex analytics. In addition, during the past 15 years, the “analytical IQ” of many organizations has evolved from reporting and dashboarding to lightweight analysis. Many are now on the verge of upping their analytical IQ by implementing predictive analytics against both structured and unstructured data. This type of analytics can be used to do everything from delivered highly tailored cross-sell recommendations to predicting failure rates of aircraft engines.
• Sustainable advantage . At the same time, executives have recognized the power of analytics to deliver a competitive advantage, thanks to the pioneering work of thought leaders, such as Tom Davenport, who co-wrote the book, “Competing on Analytics.” In fact, forward-thinking executives recognize that analytics may be the only true source of sustainable advantage since it empowers employees at all levels of an organization with information to help them make smarter decisions.
For more refer: http://docs.media.bitpipe.com/io_10x/io_101195/item_472252/Research%20report%20Chapter%201update.pdf
Subscribe to:
Posts (Atom)